Dr. Zhiwei Jiang, and his research group at our school have recently made new progress in the technique of sequence labeling: they have proposed a sequence labeling technique tailored for few-shot scenarios, which can be widely applied in sequence labeling tasks such as Named Entity Recognition and Slot Tagging.
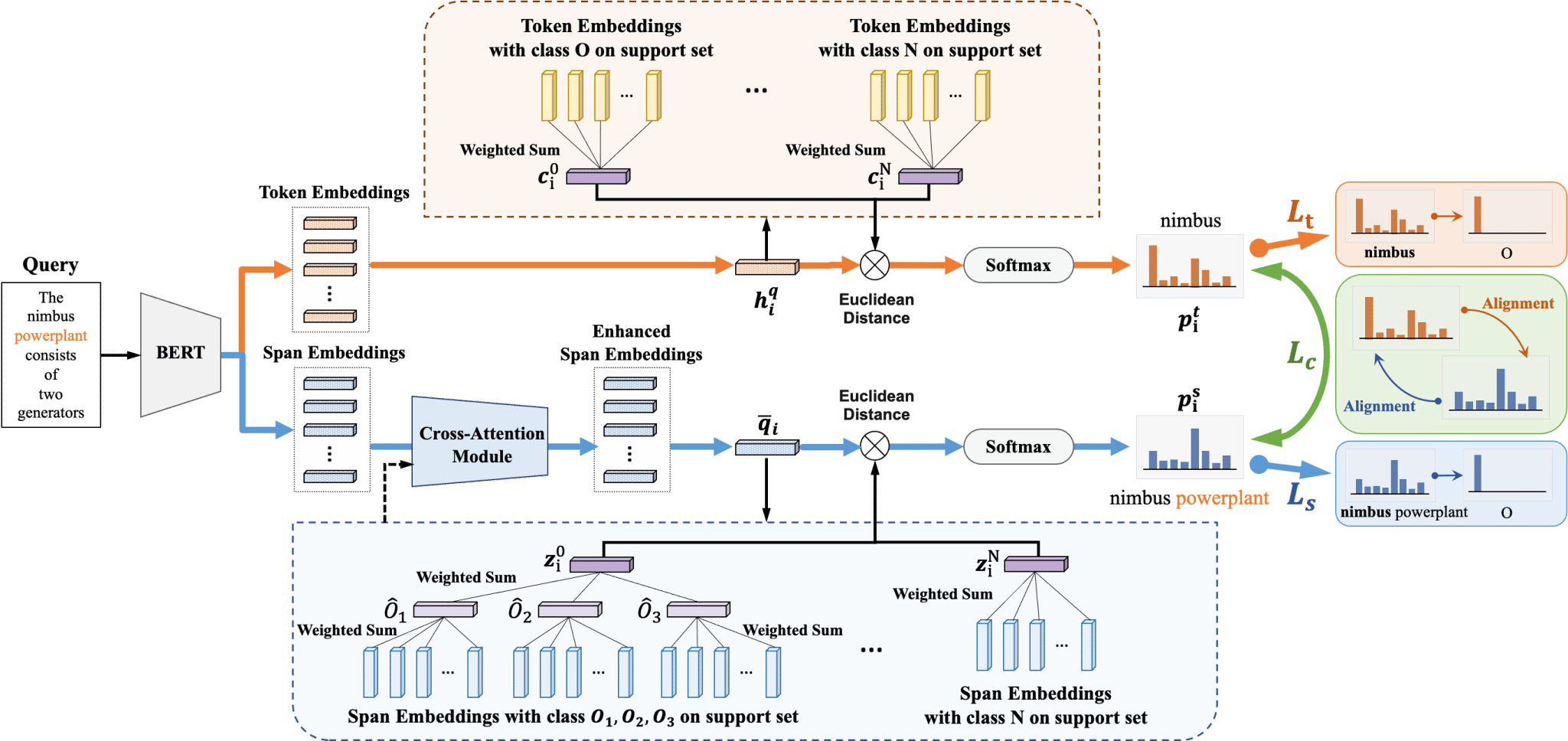
Traditional sequence labeling methods are often supervised, requiring a large amount of annotated data for training. However, this limitation restricts their application in real-world scenarios where acquiring annotated data is laborious and expensive. Few-shot sequence labeling aims to alleviate the demand for labeled data by identifying novel classes based on only a few labeled samples. Existing methods primarily address the data scarcity problem by designing token-level or span-level labeling models based on metric learning. However, these methods are typically trained at a single granularity (i.e., either token-level or span-level) and exhibit weaknesses specific to that granularity. To tackle these issues, Dr. Zhiwei Jiang's research group considers unifying token and span-level supervisions and proposes a Consistent Dual Adaptive Prototypical (CDAP) network for few-shot sequence labeling. The CDAP network comprises token- and span-level networks jointly trained at different granularities. To align the outputs of the two networks, this work further introduces a consistent loss to facilitate mutual learning. Additionally, during the inference phase, this work designs a consistent greedy inference algorithm that first adjusts the predicted probabilities and then greedily selects non-overlapping spans with the maximum probability. Extensive experiments demonstrate that the proposed model achieves new state-of-the-art results on three benchmark datasets.
The related research paper titled "Unifying Token- and Span-level Supervisions for Few-shot Sequence Labeling" has been published at the top-tier international journal in the field of information retrieval, ACM Transactions on Information Systems (TOIS2024, CCF-A journal). Students and academic peers interested in this research are welcome to email for further discussion: jzw@nju.edu.cn.