Dr. Zhiwei Jiang and his research group at our school have recently made some new progress in multimodal video understanding: (1) For the task of Audio-Visual Event Localization in videos, they propose a conditional dual-branch paradigm based on event localization preference learning. This paradigm establishes a conditional dependency relationship between event localization and event classification sub-tasks, allowing event localization to more fully utilize location preferences specific to event categories, thus achieving better event localization results. (2) For the task of Short Video Ordering, they propose a short video ordering framework based on position decoding and successor prediction. Besides, they have also meticulously curated a dedicated short video ordering dataset to facilitate the development and evaluation of new algorithms.
The two research works are as follows:
1. Learning Event-Specific Localization Preferences for Audio-Visual Event Localization
Audio-Visual Event Localization (AVEL) aims to locate events that are both visible and audible in a video. Existing AVEL methods primarily focus on learning generic localization patterns that are applicable to all events. However, events often exhibit modality biases, such as visual-dominated, audio-dominated, or modality balanced, which can lead to different localization preferences. These preferences may be overlooked by existing methods, resulting in unsatisfactory localization performance. To address this issue, this work proposes a novel event-aware localization paradigm, which first identifies the event category and then leverages localization preferences specific to that event for improved event localization. To achieve this, this work introduces a memory-assisted metric learning framework, which utilizes historic segments as anchors to adjust the unified representation space for both event classification and event localization. To provide sufficient information for this metric learning, this work designs a spatial-temporal audio-visual fusion encoder to capture the spatial and temporal interaction between audio and visual modalities. Extensive experiments on the public AVE dataset in both fully-supervised and weakly-supervised settings demonstrate the effectiveness of the proposed approach.
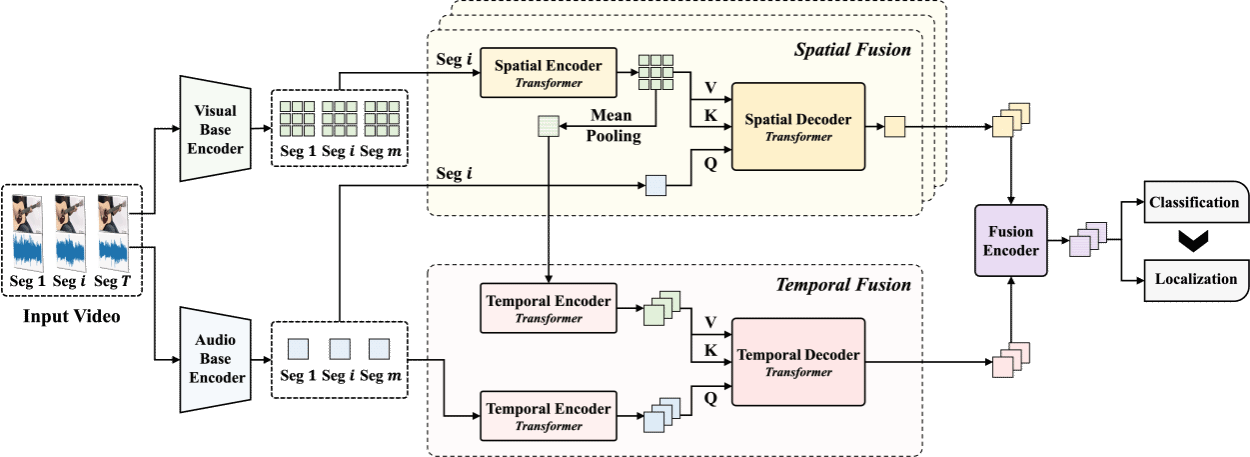
The related research paper titled "Learning Event-Specific Localization Preferences for Audio-Visual Event Localization" has been published at the top-tier international conference on multimodal processing, The 31st ACM International Conference on Multimedia (MM2023, CCF-A conference). Students and academic peers interested in this research are welcome to email for further discussion: jzw@nju.edu.cn.
2. Short Video Ordering via Position Decoding and Successor Prediction
Short video collection is an easy way for users to consume coherent content on various online short video platforms, such as TikTok, YouTube, Douyin, and WeChat Channel. These collections cover a wide range of content, including online courses, TV series, movies, and cartoons. However, short video creators occasionally publish videos in a disorganized manner due to various reasons, such as revisions, secondary creations, deletions, and reissues, which often result in a poor browsing experience for users. Therefore, accurately reordering videos within a collection based on their content coherence is a vital task that can enhance user experience and presents an intriguing research problem in the field of video narrative reasoning. In this work, Dr. Zhiwei Jiang and his research group curate a dedicated multimodal dataset for this Short Video Ordering (SVO) task and present the performance of some benchmark methods on the dataset. In addition, they further propose an advanced SVO framework with the aid of position decoding and successor prediction. The proposed framework combines both pairwise and listwise ordering paradigms, which can get rid of the issues from both quadratic growth and cascading conflict in the pairwise paradigm, and improve the performance of existing listwise methods. Extensive experiments demonstrate that the proposed method achieves the best performance on their open SVO dataset, and each component of the framework contributes to the final performance.
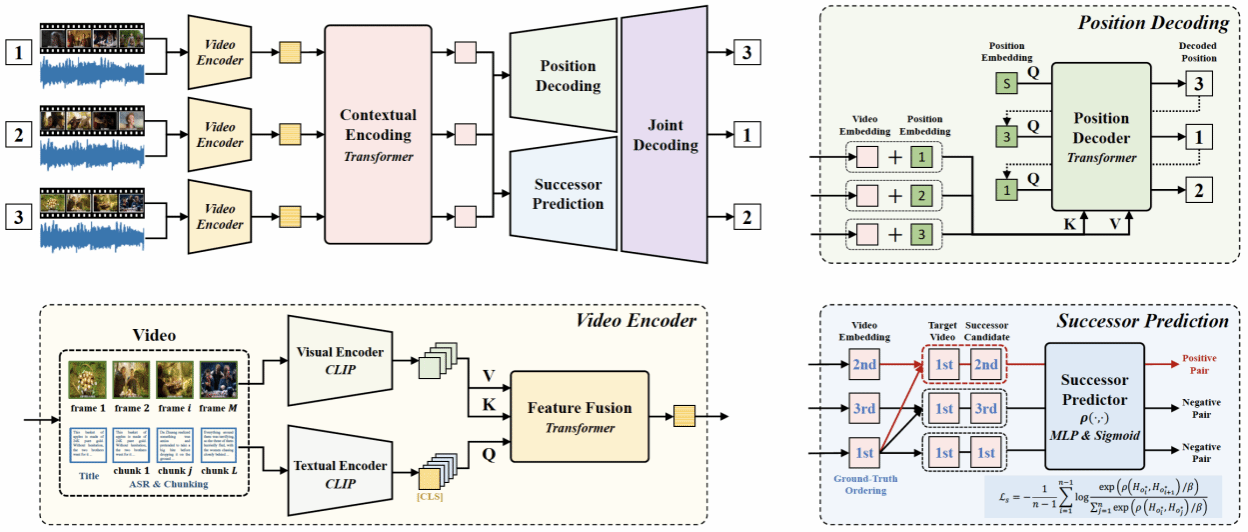
The related research paper titled "Short Video Ordering via Position Decoding and Successor Prediction" have been accepted as a full paper at the top-tier international conference in the field of information retrieval, The 47th International ACM SIGIR Conference on Research and Development in Information Retrieval (SIGIR2024, CCF-A conference). Students and academic peers interested in this research are welcome to email for further discussion: jzw@nju.edu.cn.